Autonomous vehicles (AVs) represent a revolution in transportation, with self-driving technology enabling cars to operate with minimal human intervention. These vehicles rely on advanced sensing, processing, and decision-making systems to detect and respond to real-time traffic conditions effectively. Understanding how AVs manage this complex task can shed light on the promise and challenges of self-driving technology.
Key Takeaways
- Autonomous vehicles use a combination of Lidar, Radar, cameras, and ultrasonic sensors to detect their surroundings and traffic conditions.
- AI and machine learning process sensor data, allowing the vehicle to recognize objects, predict behaviors, and make real-time decisions.
- Autonomous systems face challenges in adverse weather, complex urban settings, and ethical dilemmas, which developers are working to address.
- Emerging technologies like 5G and V2V/V2I communication are expected to enhance detection and response capabilities in future autonomous vehicles.
Sensors in Autonomous Vehicles: The Foundation of Detection
Autonomous vehicles rely on an array of sensors to perceive their surroundings. Each sensor type offers specific capabilities that contribute to a comprehensive understanding of the environment. The most common types of sensors include:
- Lidar (Light Detection and Ranging): Lidar uses lasers to map the vehicleโs surroundings in three dimensions. By emitting light pulses and measuring the time it takes for them to return after hitting an object, Lidar can create a highly accurate 3D map. This technology excels in detecting obstacles, lane markings, pedestrians, and other vehicles in real-time.
- Radar (Radio Detection and Ranging): Radar uses radio waves to detect the speed and distance of objects. Radar is especially useful for identifying other vehicles and understanding their speed and trajectory, which is vital for adaptive cruise control and collision avoidance.
- Cameras: Multiple cameras are placed around the vehicle to provide a visual understanding of the environment. Cameras detect objects like traffic lights, road signs, lane markings, pedestrians, and other vehicles. They are crucial for recognizing colors and textual information that Lidar and Radar cannot detect.
- Ultrasonic Sensors: Ultrasonic sensors detect objects that are close to the vehicle, such as curbs, walls, or other vehicles during low-speed maneuvers. These sensors help with parking and obstacle detection in confined spaces.
Processing Data Through AI and Machine Learning
The data collected by these sensors is processed by an onboard computer, which uses advanced algorithms and machine learning models to interpret and respond to traffic conditions. Key components in data processing include:
- Object Detection and Classification: AI models analyze the data from sensors to identify various objects, distinguishing between pedestrians, vehicles, bicycles, and stationary obstacles. Machine learning enables the system to improve its recognition accuracy over time as it encounters diverse situations.
- Prediction Models: After identifying objects, autonomous vehicles use prediction models to estimate the future behavior of moving objects. For instance, the vehicle may anticipate a pedestrianโs movement to determine if they are likely to cross the street. Predictive algorithms play a crucial role in understanding the intentions of other drivers and pedestrians.
- Path Planning and Decision-Making: Based on the detected objects and predictions, the vehicle determines the optimal path. This process involves planning a safe route that avoids obstacles, obeys traffic laws, and adapts to changing traffic conditions. The system assesses factors like speed, direction, and the distance between the vehicle and other objects.
- Real-Time Updates: To ensure safety and efficiency, the onboard computer processes data in real-time. This rapid processing is vital for responding to sudden changes, such as a vehicle suddenly stopping or a pedestrian stepping onto the road.
Responding to Traffic Conditions
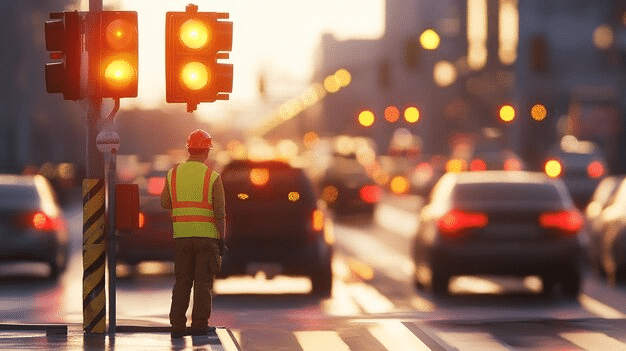
After analyzing the environment and planning a safe path, the vehicleโs control system executes appropriate actions to navigate safely. Autonomous vehicles respond to traffic conditions in several ways:
- Adapting to Traffic Flow: By analyzing the speed and behavior of nearby vehicles, autonomous cars adjust their speed and position accordingly. For instance, in heavy traffic, the vehicle may reduce its speed and increase its following distance to ensure safety.
- Obstacle Avoidance: When an obstacle is detected, the vehicle may slow down, stop, or change lanes to avoid it. Advanced algorithms help the car to decide the best way to navigate around stationary or moving obstacles, such as construction cones, vehicles, or pedestrians.
- Following Traffic Signals and Road Signs: Cameras recognize traffic lights and signs, allowing the vehicle to stop at red lights, yield, and follow speed limits. Autonomous systems also interpret lane markings to maintain proper lane positioning, even when road markings are faded or obscured.
- Emergency Maneuvers: If an unexpected situation occurs, such as a sudden stop by the car in front, the vehicle can perform emergency braking or evasive maneuvers. These responses are often faster than human reflexes, contributing to improved safety.
Challenges in Detecting and Responding to Traffic Conditions
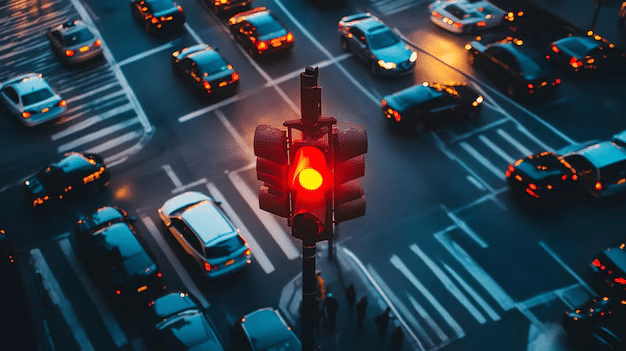
While autonomous vehicles offer remarkable capabilities, they face several challenges in detecting and responding accurately to traffic conditions:
- Weather Conditions: Rain, fog, and snow can affect the performance of sensors, particularly cameras and Lidar. In such conditions, visibility is reduced, and reflections can distort sensor readings, impacting the vehicleโs ability to detect objects accurately.
- Complex Urban Environments: Navigating crowded city streets with unpredictable pedestrian behavior, cyclists, and frequent intersections requires sophisticated algorithms. Urban environments present challenges such as unmarked pedestrian crossings, jaywalking, and parked vehicles, which can confuse the system.
- Construction Zones and Roadwork: Construction areas often lack clear markings and include temporary barriers, cones, or workers in unusual positions, making it difficult for autonomous systems to navigate safely. Some systems struggle to distinguish between temporary and permanent objects.
- Ethical Decision-Making: Autonomous vehicles sometimes face ethical dilemmas where they must choose between two unfavorable outcomes. For example, if a pedestrian unexpectedly steps in front of the vehicle, it may need to decide between swerving into another lane or performing emergency braking, potentially endangering other drivers or passengers.
The Future of Autonomous Vehicle Detection and Response
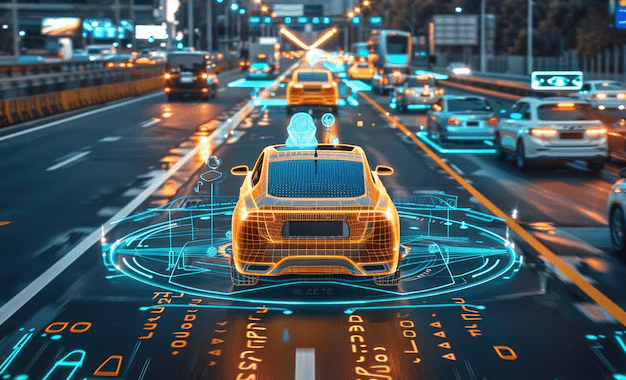
As technology advances, improvements in sensor technology, AI, and machine learning are expected to address some of these challenges. Increased reliance on 5G networks, vehicle-to-vehicle (V2V) communication, and vehicle-to-infrastructure (V2I) communication could further enhance AVsโ ability to detect and respond to traffic conditions. By connecting with other vehicles and infrastructure, autonomous cars can receive real-time data on traffic conditions, construction zones, and road hazards beyond the range of their onboard sensors.
Also Read : How Does Cyber security Ensure Data Protection In Cloud Computing?
Conclusion
Autonomous vehicles are at the forefront of transportation technology, using advanced sensor arrays and AI-driven processing to detect and respond to traffic conditions. While Lidar, Radar, cameras, and ultrasonic sensors allow these vehicles to gather a detailed view of their environment, machine learning and predictive algorithms help translate this information into safe, efficient navigation. Despite impressive advancements, challenges remain, particularly with adverse weather, complex urban landscapes, and construction zones. However, future enhancements in connectivity, such as 5G and vehicle-to-infrastructure communication, promise to bridge these gaps and lead to safer, more responsive autonomous driving. As the technology progresses, the journey toward fully autonomous vehicles that navigate seamlessly among human drivers and respond to all conditions becomes increasingly attainable, making self-driving cars a realistic part of tomorrow’s transportation landscape.
FAQs
How do autonomous vehicles know when to stop?
Autonomous vehicles use cameras and Lidar to detect traffic lights and stop signs. When these signals are identified, the vehicleโs control system applies the brakes accordingly.
What happens if an object suddenly appears in front of an autonomous vehicle?
If an object suddenly appears, the vehicleโs emergency detection system will initiate evasive actions, such as braking or swerving, depending on the situation.
Can autonomous vehicles detect pedestrians and cyclists?
Yes, AVs use Lidar, Radar, and cameras to identify pedestrians and cyclists. AI algorithms predict their behavior, allowing the vehicle to take preventive actions if needed.
Do weather conditions affect the ability of autonomous vehicles to detect traffic conditions?
Adverse weather like rain, snow, or fog can affect sensor performance, especially for cameras and Lidar, making it harder for the vehicle to detect objects accurately.
How do autonomous vehicles respond to construction zones?
Some autonomous systems struggle with construction zones due to temporary objects, unclear markings, and irregular layouts. However, advanced systems rely on mapping and real-time data to navigate these areas safely.